Nan Zhang's Blog
Friday, November 27, 2020
Hello Radio: How to Listen to FM Radio Using LimeSDR
Tuesday, November 24, 2020
Signal Processing Magic (7) -- Doppler Shift, Rayleigh fading and Jakes model
Doppler shift was discovered by a physicist named Christian Doppler long time ago. Doppler shift is used to characterize the frequency change due to movement. A typical example is that when a fire truck drives toward you, the tone of its siren changes over the time because its speed towards you varies.
Doppler shift for wireless communication is
\[f_d=v/c*f_c\]
whereas v is the speed of the object, c is the speed of light, and \(f_c\) is carrier frequency. For instance, if you sit on a high-speed training moving at 350km/h browsing Internet and your phone is using a 3GHz band, then
\[f_d = (350*1000/3600)/(3*10e8)*3*10e9 = 972 Hz\]
Based on this equation, moving faster as well as using a band with higher carrier frequency will make Doppler shift larger. Usually the highest Doppler shift is observed in high-speed train. Larger Doppler shift makes channel estimation harder in wireless communication.
Rayleigh fading is a commonly used model for a wireless channel, and it is used when the transmitter can't see the receiver such as in Manhattan island. Rayleigh fading model can be derived from joint Gaussian distribution. Assuming \(x\) and \(y\) are two zero-mean Gaussian R.V. orthogonal to each other, their joint distribution becomes:
\[f_{X,Y}(x,y)=\frac{1}{2\pi\sigma^{2}}e^{-\frac{x^2+y^2}{2\sigma^2}}\]
Rayleigh fading tracks the envelope, \(r\). With \(r^2=x^2+y^2\), we have \(x=r*cos(\theta)\) and \(y=r*sin(\theta)\). To transfer a \(f_{X,Y}(x,y)\) to distribution of \(r\), the determinant of the Jacobian matrix needs to be found:
\[J(r,\theta)=det\begin{bmatrix}\frac{\partial x}{\partial r}&\frac{\partial x}{\partial \theta}\\\frac{\partial y}{\partial r}&\frac{\partial y}{\partial \theta}\end{bmatrix}=det\begin{bmatrix}cos(\theta)&-r*sin(\theta)\\sin(\theta)&r*cos(\theta)\end{bmatrix}=r\]
Therefore,
\[f_{R,\Theta}(r,\theta)=\frac{J(r,\theta)}{2\pi\sigma^{2}}e^{-\frac{r^2}{2\sigma^2}}=\frac{r}{2\pi\sigma^{2}}e^{-\frac{r^2}{2\sigma^2}}\]
After integrating over \(2\pi\) to remove \(\theta\), Rayleigh fading model is:
\[f_{R}(r)=\frac{r}{\sigma^{2}}e^{-\frac{r^2}{2\sigma^2}}\]
for \(r\geq0\).
In reality, fading channel is always correlated in time. That means the wireless channels your phone sees in this moment and the next moment are always more or less similar. Fourier transmission of wireless channel's time-domain auto-correlation becomes its Power Spectral Density (PSD). For Rayleigh fading with vertical antenna and signal coming from all angles in uniform distribution, it has a famous PSD called Jakes model. Jakes model also depends on Doppler shift, and it is:
\[S(f)=\frac{1}{\pi f_d}\frac{1}{\sqrt{1-(f/f_d)^2}}\]
when \(|f|\leq f_d\) and \(S(f)=0\) otherwise. Jakes model has a famous U shape and the width of the "U" depends on Doppler shift, \(f_d\). When \(f_d=0\), Jakes model is an impulse and it means Rayleigh channel never changes.
Low Light Image Combining Using Python (3) -- Running Even Faster
Saturday, April 4, 2020
Low Light Image Combining Using Python (2) -- Running Faster
Baseline: 155.8s
After using decimated image for alignment: 40.6s
After using decimated image for alignment + processing in multi-core: 21.1s
Now let me show you how to do it:
Using decimated image for alignment
Image alignment is the most computationally intensive part of the image combining pipeline. In fact, the time spent on image alignment is more than 90% of the total. The operation of image alignment is shown below. During image alignment, the candidate block is swiped though the target block in both horizontal and vertical directions. Assume block size is B, search range in both horizontal and vertical are S, the complexity of image alignment is proportional to B*S*S. For a 4k x 3k image and search range equal to 40 ([-40,40] with each even position), the complexity for matching each pair is 19.2G operations, which is enormous.
One observation is that due to the structure of Bayer pattern, we only calculate candidate of even number of pixel shift such as 0/2/4 etc. Then a natural thought is to decimate both candidate and target by 2. This will bring ~4x acceleration. Instead of B*S*S complexity, it is now B*S*S/4. This explains why processing time is reduced from 155.8s to 40.6s after decimation. Performance wise, this decimation means that instead of using pixels of all colors, only one out of four pixels with green color will be used for alignment. However, the quality of combining seems to hold, which indicate that there are enough pixels left to guarantee the quality of image alignment. The Python code change is below, ":2" is the delta:
Old code:
candidate = rgb_raw_image_candi[f,row_start+row_offset+boundary_adjust[row, col, 0]:row_end+row_offset+boundary_adjust[row, col, 1], col_start+col_offset+boundary_adjust[row, col, 2]:col_end+col_offset+boundary_adjust[row, col, 3]] target = rgb_raw_image[row_start+boundary_adjust[row, col, 0]:row_end+boundary_adjust[row, col, 1], col_start+boundary_adjust[row, col, 2]:col_end+boundary_adjust[row, col, 3]]
New code:
candidate = rgb_raw_image_candi[row_start+row_offset+boundary_adjust[row, col, 0]:row_end+row_offset+boundary_adjust[row, col, 1]:2, col_start+col_offset+boundary_adjust[row, col, 2]:col_end+col_offset+boundary_adjust[row, col, 3]:2] target = rgb_raw_image[row_start+boundary_adjust[row, col, 0]:row_end+boundary_adjust[row, col, 1]:2, col_start+boundary_adjust[row, col, 2]:col_end+boundary_adjust[row, col, 3]:2]
Multi-core processing
Our baseline script use single thread to process. By distributing tasks to multiple threads, we expect the running time to be shorter. Introduction of Python-based parallel processing can be found here. Our task is to align three images with the base image. Therefore, it can be divided into three sub-tasks which are to align each image with the base. In this way, these three sub-tasks can be run independently which brings the best parallel processing gain. Since the sub-tasks are independent, we use the most basic parallel processing module of pooling. What pooling module does is to assign each sub-task to a thread. image_align is the function for sub-task execution and image_input is a list with each element to be input images for a sub-task.
Pooling code:
with Pool(6) as p: image_output = p.map(image_align, image_input)
Due to the overhead of parallel processing, the processing time is not cut to 1/3 of single thread. However, parallel processing does reduce the running time by ~20s (40.6s -> 21.1s). In general, when each sub-task is more computationally heavier, there is more time saving by parallel processing.
With both alignment with decimated image and multi-core processing, the final processing time is reduced to 21.1s from 155.8s. My code can be found here
Sunday, March 29, 2020
Low Light Image Combining Using Python (1)
The pipeline includes three steps: raw image alignment, raw image combining, post processing.
Raw image is the direct output of the image sensor. In normal photo, each pixel has three color channels as R/G/B. But in raw image, each pixel only has one color channel. Bayer pattern is widely used in raw image, and it is also used in this experiment. The color pattern used here is shown below which is one kind of Bayer patterns. As one can see, out of 24 pixels, half of them are green, 25% is red and 25% is blue. The reason that green channel occupies the most pixels is because human eye is the most sensitive to green color.
0 1 2 3 4 5
0 G R G R G R
1 B G B G B G
2 G R G R G R
3 B G B G B G
What raw image alignment does is to align two raw images. Since multiple images are taken at different times, due to hand movement, there will be small shift of the image. Without alignment, adding images together will create blurry photo. Our method for image alignment is straightforward. To align two images, we will divide both images to blocks with equal dimension. By calling one image "target" and the other image "candidate", we will swipe the candidate block through the target block in both x and y axis search directions. The scope of the swipe is called search range. For each swipe position, the L1 distance will be calculated which is the delta of the pixel values of the candidate and target blocks. Out of all swipe positions, the one with smallest L1 distance will be selected. For more details of this operation, you can refer to the Python source code here.
The reason that image alignment should be done at the very beginning of the image pipeline on raw image is for both performance and computation time. In term of performance, additional processing of the signal at the later stages such as interpolation/correlation may degrade the performance of alignment. In term of computation time, raw image has one color in a pixel but at later stages after interpolation, there will be three colors in each pixel. Calculating alignment on one color channel is faster than calculating that on three channels. Due to the structure of Bayer pattern, we only calculate candidate of even number of pixel shift such as 0/2/4 etc.
The step of raw image combining is to add candidate and target blocks together. Since they are already aligned, adding them together will enhance the signal while reducing the noise. Here we apply equal weight to each image. However, performance could be improved by applying different weights.
Between raw image and the photo we usually see, post processing needs to be done. Libraw library is used for post processing. The major steps of post processing is shown below:
Next we will show the outcome of combining. Raw images are obtained using Pro camera mode of a Samsung Galaxy S9 device. The camera settings are ISO = 800, 1/4 shutter speed, and F1.5. S9 has two aperture modes: F2.4 and F.15. F1.5 is a larger aperture for low light photo. Figure below shows the difference before (left) and after (right) the combining. The image quality is clearly improved. To get the right figure, we combine eight low light images. Bilateral filtering can be used to further enhance the image quality.
Source code of the Python script and raw image files can be found here: https://github.com/legendzhangn/blog/tree/master/lowlight_image_combine
Sunday, February 16, 2020
LibRaw Post Processing Pipeline Translated Into Python (2) -- Low Light Photo
Then we found that compared with LibRaw, our Python script does not work well for raw image taken under low light. While LibRaw still shows the objects albeit quite noisy, our original Python script does not show anything. After some digging, it is found that LibRaw has an auto bright function which is not available in the previous Python script. As shown below, for low light image, the difference made by using auto bright function is quite stark.
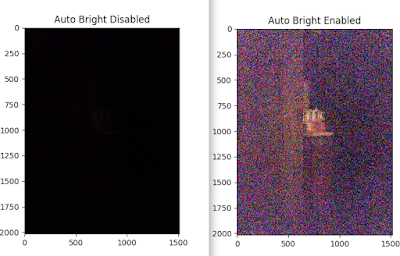
Thereafter, our Python script gets updated and now it supports auto bright as well. The main difference between low light image and normal light image is how Gamma mapping is done. For low light image, Gamma curve peaks much faster than for normal light image. The exact Gamma curve of low light image depends on its histogram. The updated script can be found here, and it shows how this new Gamma curve is generated.
Monday, December 30, 2019
An Android App for Photo Brightening
To use the app, the GUI has three buttons: Load, Save and Setting. "Load" button can load photo from Android gallery. After loading, post processing immediately starts. Post processing takes 10-30 seconds depending on the image size. It runs slower than some of the similar apps but produces better results. "Save" button allows to save the results. Note that to enable saving, the storage permission needs to be enabled in Android setting for this app.
This Youtube video shares how to use the app.
Hope that this introduction is useful and you can enjoy using this app. Any feedback is welcomed.